Data scientists vs. data analysts: Who's who?
It all started in 1985. Microsoft brought to market a new tool that would revolutionize the way companies do business, enabling them to collect, organize, and understand their information better than ever before, and spawning a new discipline called business analytics. You know what we are talking about, don't you? That is right: the well-known Excel.
Over the following thirty years, data analysis, along with advances in technology and computing, has become a powerful weapon for businesses looking to learn more about their customers and differentiate themselves from the competition. Today, it is impossible to talk about growth, optimization, or differentiation without talking about the use of data within companies.
Data analytics is here to stay, and it is not stopping anytime soon. This is why new professionals have emerged who master this field and can understand data and extract value from it. However, as the sector and technology advance, the issue becomes more complicated since numerous profiles and experts seem to all do the same type of tasks. This occurs, for example, with data scientists and data analysts.
Whether you are a student deciding on a career path or an employer looking to hire someone with data talent, one of the most common questions is what is the difference between a data scientist and a data analyst. Who is who? Who does what? Don't they both deal with computers, numbers, algorithms, mathematics, statistics, and tables non-stop? Well, that is kind of true. But the reality is that both have very different profiles, and in this article we are going to learn about their differences.
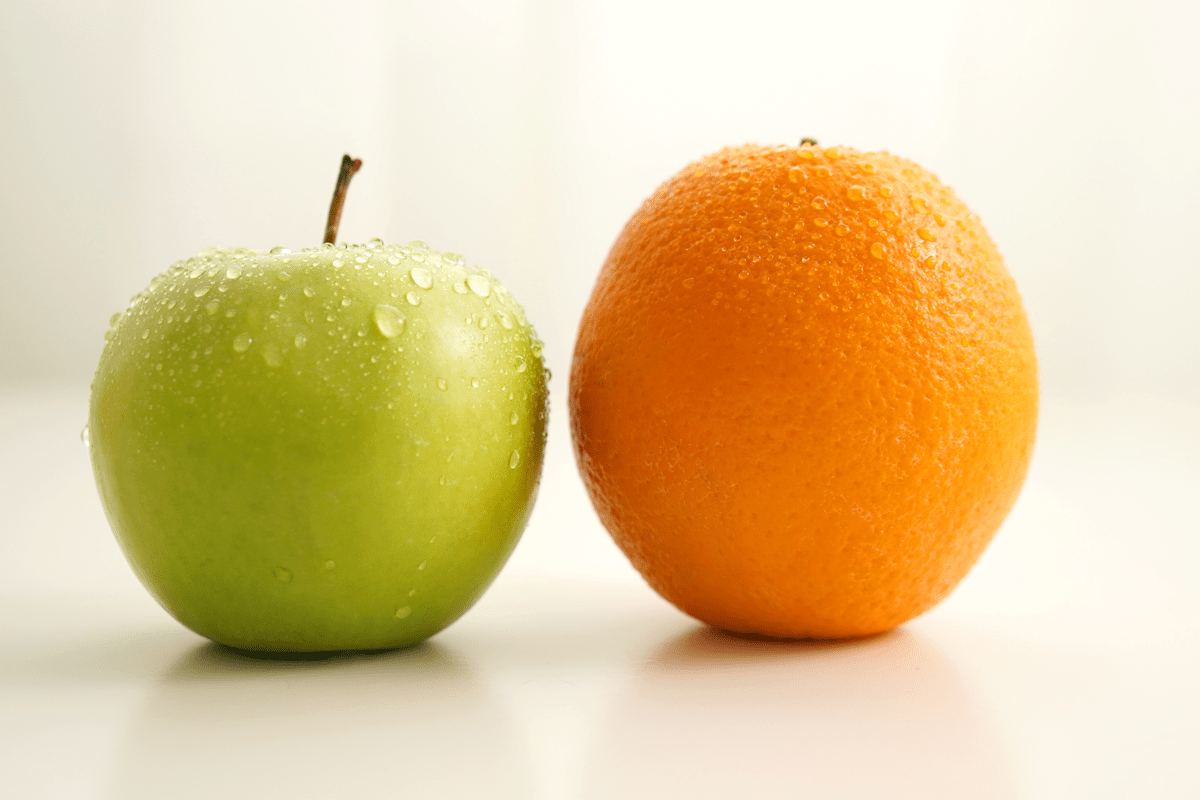
Data scientists: When did they appear?
The term “data scientist” officially emerged in the last decade, but that does not mean that no one was doing a similar job more than ten years ago or that a more experienced data scientist did not exist. For example, many of those who started in fields such as statistics, actuarial science, or quantitative analytics are undoubtedly doing work that overlaps with today's data scientists, and some have even made the full leap into data science. In the same way, they may also have training in areas of study such as computer science, and many (about half) have doctoral degrees. Nowadays, the importance given to data science or data education courses is increasing, as is the discussion of what qualifies someone as a data analyst or data scientist.
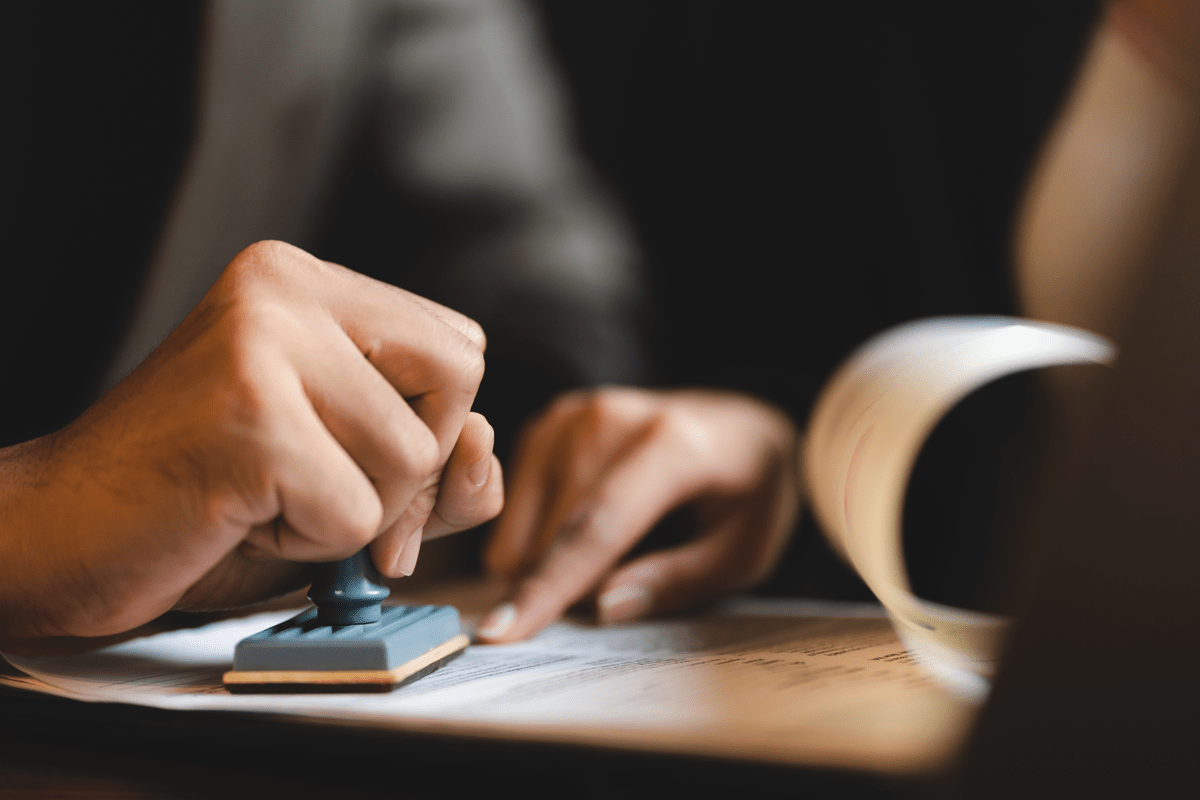
In the case of data analysts, they cover various areas of study and specialization, from business and finance to training in the liberal arts. But the delineation is further complicated by the rise of the so-called "citizen data scientist", who seems to merge the two worlds.
So, is it a specific area of study or learning a skill that pushes a data analyst to become a data scientist? Or is it the other way around due to a lack of knowledge in a specific area? The answer is no, especially considering that a certain experience or skill (for one or the other) may be important on paper in one company or industry but not in another.
Let's talk about their skills
One of the most common characteristics used to distinguish data scientists from data analysts is that the former focuses on more technical practices. However, it is worth noting that while data scientists are generally more proficient in coding environments, there are plenty of data analysts who are also technical and comfortable with that language.
It is also important to note that data scientists who have all the technical skills in the world will not do a good job if they don't also have communication skills and/or business vision. That is the ability to connect with those who know the business best and use those technical skills to make a real impact.
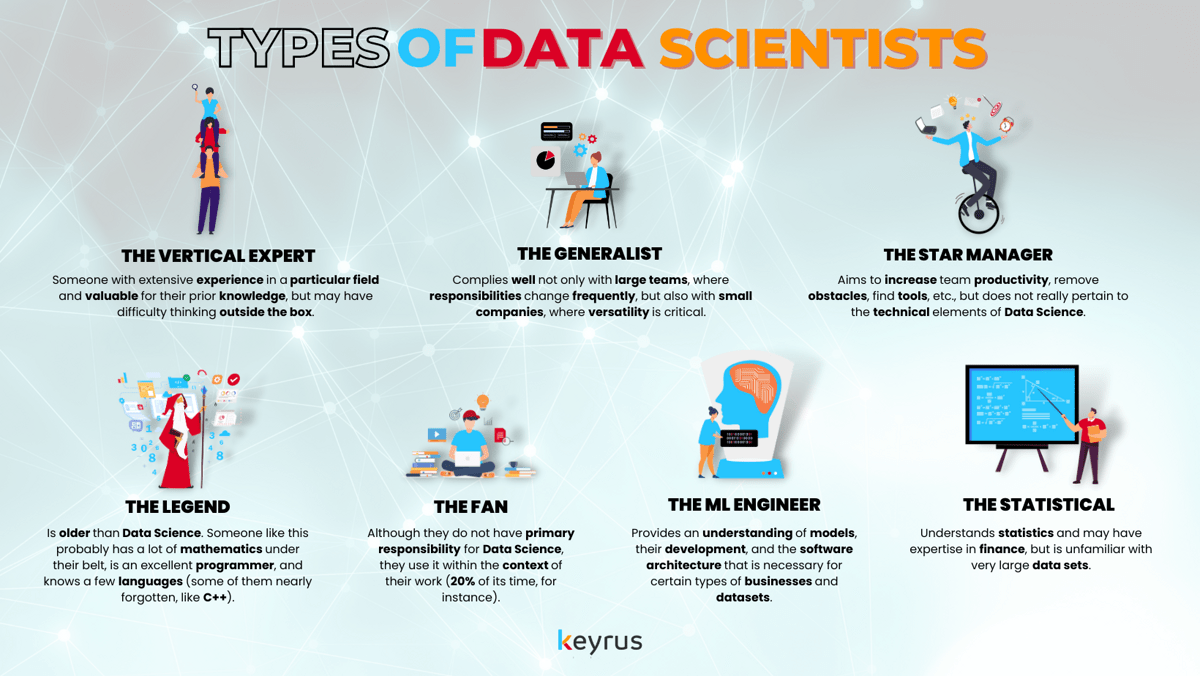
With the rise of machine learning, data analysts and other professionals who lack in-depth knowledge of machine learning and statistics are scrambling to expand their usefulness in the data chain. But these advances will not make data scientists obsolete either. Their capabilities around machine learning interpretability and model maintenance are generally areas that business or data analysts do not master.
And if we talk about common skills for the two profiles, it is worth mentioning data cleaning and exploration since they are probably the only two (along with the ability to question data and link data projects to business needs) that are critical for both data scientists and analysts. And that is because most of the data projects consist of getting the information right (that is, the preparation phase, which is the one that takes the most time) so that machine learning (or whatever the goal) can then be applied, so those two skills become the cornerstone of both positions.
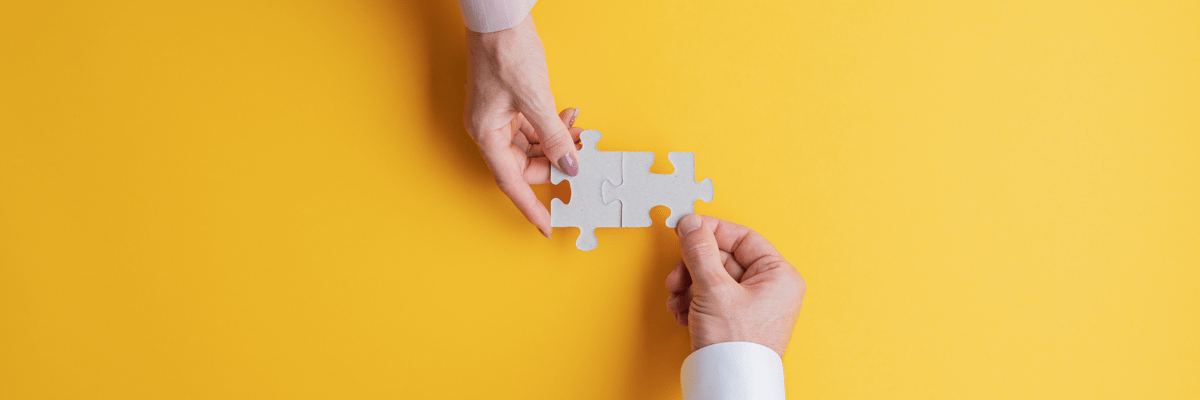
But not everything is black and white
Companies looking to hire one of the two positions should take a critical look at their current staff, their needs, and the ultimate goal of the data team or data projects. Hiring someone who will ultimately produce dashboards for internal use will require an entirely different skill set than hiring someone whose KPI is putting a machine learning-based fraud detection model into production.
The right tools can also elevate your staff, making them more efficient and productive, whether they are data scientists or data analysts. For example, data science, machine learning or AI platforms can help an organization work together across all domains by closing the loops between business people, data analysts, data scientists and IT or data engineers.
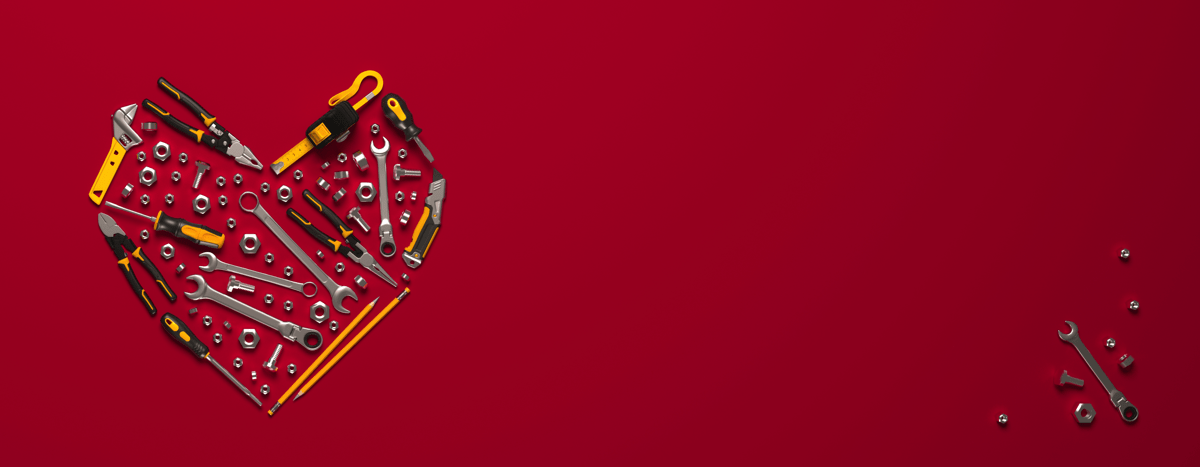
Ultimately, much like education, the skills needed to be a data scientist vs. a data analyst are not black and white. There is a lot of overlap between the two profiles and depending on the needs and objectives of each company, it will be assessed which of the two is a better fit in that particular case.
Are you ready to make a difference with your data expertise?
Join Keyrus and be a part of a team of over 3500 experts with decades of experience delivering data projects to clients across the globe. At Keyrus, you will have the opportunity to work with cutting-edge technologies and tackle real-world challenges that drive impact and innovation.
Whether you are passionate about data analysis, data science, or any other aspect of the data landscape, you will find a supportive and dynamic environment where you can grow and excel. So why wait? Discover the limitless possibilities of a career at Keyrus today and unleash your full potential. Visit our list of jobs to learn more!